On February 17, ZIBS "China+" series seminar "Alternative Data and Credit Assessment" was successfully held online. Sidharth Kamani, the senior credit risk professional at the New Development Bank, shared his views on alternative data and credit assessment. Dasol Kim, ZIBS Global Cooperation Manager, hosted the lecture and led a wide-ranging discussion on topics such as the impact of artificial intelligence (AI) and electronic payment platforms on alternative data and credit assessment.
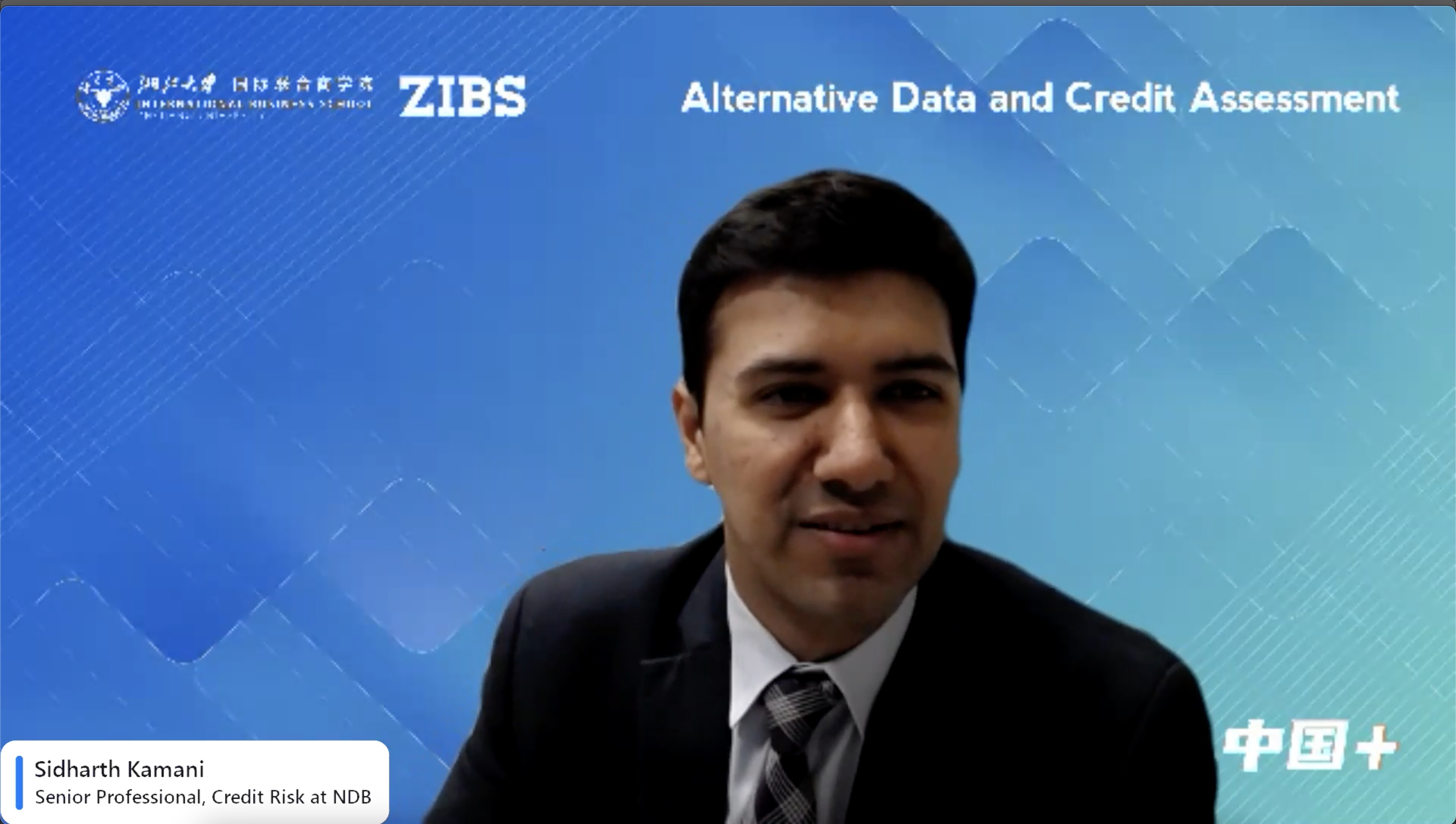
Sidharth Kamani first explained the definition of credit assessment. Credit assessment is the process of evaluating the credit risk of individuals or businesses. This typically involves collecting and analyzing information related to credit, such as credit history, income, debt, repayment records, etc., and determining a person's or business's creditworthiness. Credit assessment is commonly used to determine whether to provide credit products such as loans, credit cards, and lease agreements and to establish corresponding rates and conditions.
He mentioned that in the past few years, due to the acquisition of data by machine learning and AI, credit assessment has undergone a transformation. The acquisition of alternative data is changing the way banks assess creditworthiness, allowing them to serve a broader range of customers. With the emergence of mobile banking and machine learning, banks can optimize credit scores, thereby enhancing their ability to issue loans. This has enabled previously underserved populations to achieve financial inclusion.
Sidharth Kamani used companies such as Alibaba and Tencent in China and Amazon, Google, and Apple in the United States as examples to illustrate the growth pattern followed by alternative data. These companies create an e-commerce platform, followed by a payment platform to provide loans, and then sell insurance and savings products. The model is effective because the e-commerce platform allows companies to use data to create the platform and additional data on the payment platform to evaluate creditworthiness. From here, the ability to access and analyze data has changed the credit assessment process.
Meanwhile, alternative data scoring has completely changed the credit scoring of the unbanked population. The unbanked population, which refers to those who have never received a loan or credit card, is invisible to the banking system. This also leads to higher rates and limited access to financial products for this segment of the population. However, alternative data scoring is changing this situation by providing a way to evaluate creditworthiness for those who are coming to the bank for the first time.
Sidharth Kamani mentioned that Alipay is an example of a company that uses alternative data. It even provides credit scores for people with no credit history. Alipay has a credit score for you even if you don't have a credit card or have never taken out a loan before. Mobile phone payments and bank payments made through the phone are an important source of alternative data for Alipay. It records personal financial transaction data and non-financial data such as digital footprints, social media usage, and social psychology tests. These sources allow Alipay to have a deeper understanding of individuals' payment capabilities and willingness.
Sidharth Kamani stated that the use of big data and artificial intelligence in the credit scoring industry can allow AI-based credit scoring models to quickly determine creditworthiness based on thousands of data points. Research has found that these models perform as well as, if not better than, traditional credit scoring models, especially in negative economic conditions. However, he also highlighted concerns around data privacy and ethics in the collection of digital footprints. Regulators are now focused on ensuring the responsible and ethical use of data by companies using big data and AI. In the world of alternative data and machine learning models, US regulatory agencies are encouraging the responsible use of data to expand financial inclusivity.
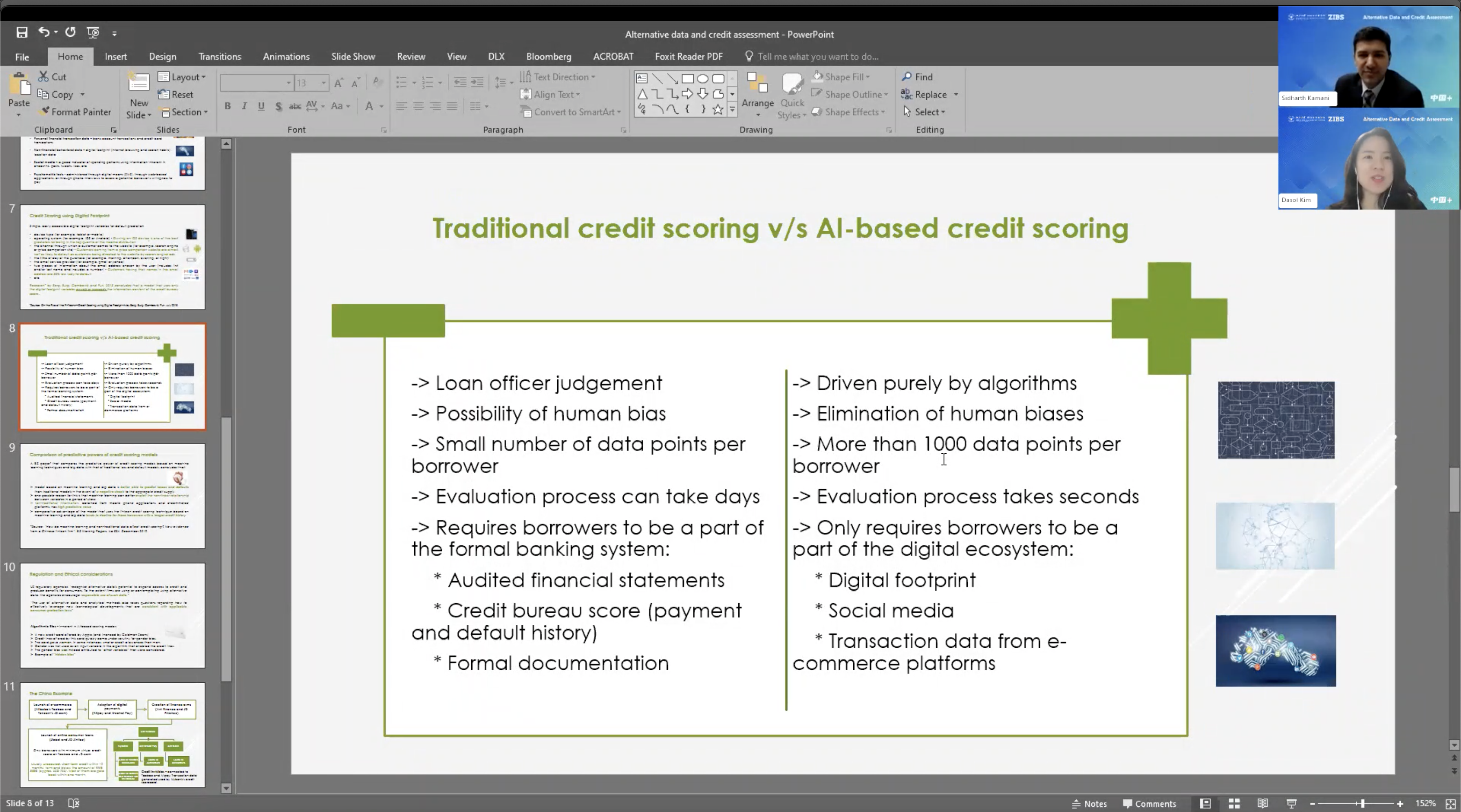
However, even with good regulation in the future, relying solely on AI-based credit scoring models cannot completely eliminate bias. An example of inherent bias can be seen in the credit card services offered by Apple and Goldman Sachs, where the cards offer women lower credit limits than men. In China, the use of alternative data allows new merchants to obtain unsecured short-term loans to build inventory and maintain their businesses. While this is a transformative change, some are concerned about the possibility of bias in the data and models used. Regulatory bodies are catching up with these issues, but there is still much work to be done to ensure the more reliable use of alternative data.
In the final sharing session, Dasol Kim discussed with Sidharth Kamani.
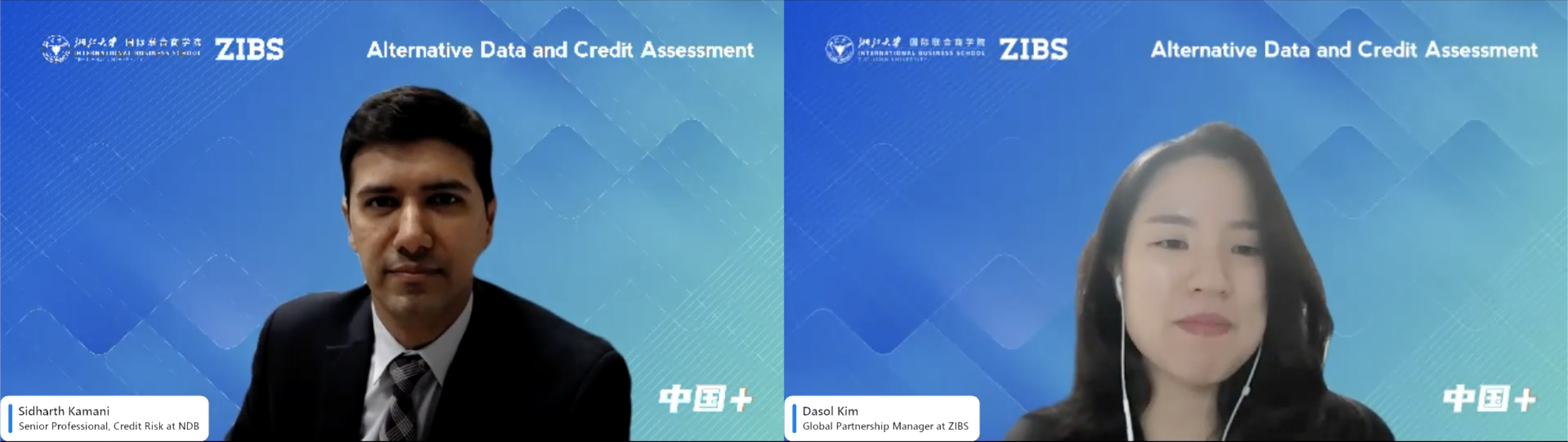
Regarding the question of how financial institutions should handle and prevent risks in credit scoring models, Sidharth Kamani emphasized that the necessity of regulation and the need to account for existing biases in data are two key areas being studied by every regulatory agency worldwide. Regarding how developing countries can improve financial inclusion, Sidharth Kamani believes that there are three crucial elements for fintech companies in developing countries to use AI-based credit scoring systems: ensuring that everyone has a bank account, a mobile phone, and internet access. It is worth noting that mobile penetration rates are increasing in almost every country in the world, making this a topic worth studying and exploring in the future.